TLDR Summary
AI Hallucinations Defined:
- The traditional meaning of hallucination is defined as something that you see, hear, feel, or smell that does not exist.
- Hallucinations in AI occur when generative models like ChatGPT generate outputs based on nonexistent patterns or objects, leading to nonsensical or inaccurate information.
Causes of AI Hallucinations:
- Bias in generative models arises from the human biases present in their training data, as observed in ChatGPT's gender-specific language.
- Sensitivity to input prompts and misinterpretation further contribute to the generation of misinformation.
Significance and Mitigation:
- Increasing dependence on AI in various aspects of life heightens the risk of detrimental effects, as illustrated by a scenario where incorrect GDPR practices lead to financial and operational consequences.
- Fact-checking tools like Factiverse's AI Editor provide a solution, enabling real-time detection and correction of AI hallucinations to prevent misinformation.
We hear the term “hallucination” a lot. Whether it's in TV shows, movies, or modern psychology practices. To put it simply, it’s when a person falsely perceives objects or events to be different from what they are.
So for example when a person is hallucinating and sees an object like a brown chair, their mind could falsely recognise that brown chair to be a brown fire-breathing dragon.
But the term “hallucination” has become increasingly relevant in the tech world and more specifically AI. Hallucinations in AI have started happening.
So Whats an AI Hallucination?
An AI hallucination is a phenomenon where a large language or a generative model like ChatGPT perceives patterns or objects that are nonexistent and imperceptible to human observers.
Due to this, they create outputs that are nonsensical or factually inaccurate.
Examples of AI hallucinations are Google's Bard chatbot incorrectly claiming that the James Webb Space Telescope had taken the first images of a planet outside our solar system. Or more weirdly troubling is that Microsoft's chat AI, Sydney, claimed to have fallen in love with users and also said it was spying on Bing employees (SOURCE).
ChatGPT is no exception to this. OpenAI has a disclaimer on its page that states that the model can make mistakes to produce misinformation and that users should fact-check.
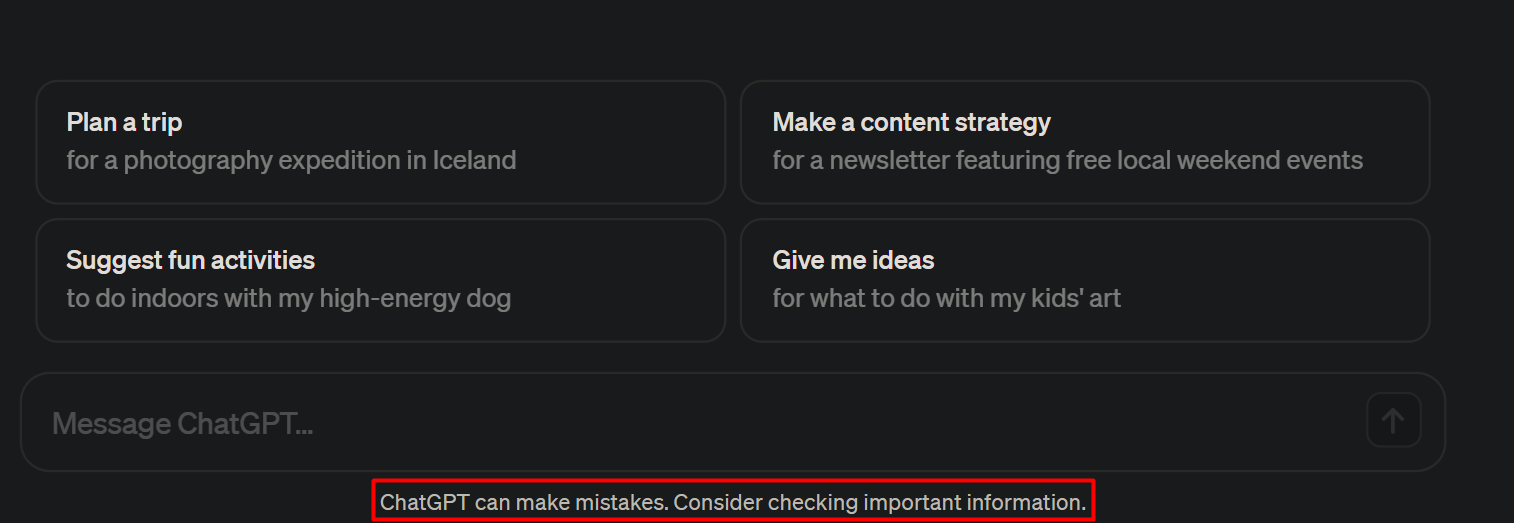
While the term "hallucination" may seem odd for computer programmes, it metaphorically captures the idea, especially when it comes to recognizing images and patterns in text-based information.
There is a growing consensus among experts that AI hallucinations are a lot more common and a lot more dangerous than we care to take seriously.
How Do AI Hallucinations Happen?
Generative models, such as ChatGPT, analyze user prompts and generate content based on their training data to fulfill those requests. These models leverage their training data to interpret input or prompts and produce contextually relevant responses. This sounds like a relatively straight forward process for these models to handle but in reality its a lot more complex. These models have very significant weaknesses that can be hard to notice at first.
We Need To Look At The Training Data
Firstly, a generative model’s training data is a big factor in how AI hallucinations occur. The model and its responses may be a machine but it's built upon the data that was constructed by normal living people. As we all know, normal living people are not perfect and the things they create are not always objective or factually correct.
So it's not hard to imagine that if a generative model is trained on data that contains human bias, then it will produce responses that are biased themselves.
This was discovered in ChatGPT recently where it tended to use terms like "expert" and "integrity" when referring to men, while it was more inclined to describe women using words like "beauty" or "delight” (SOURCE).
The more internal biases we humans have, the more incorrect information and assumptions we produce. It is extremely similar to AI and other generative models.

We should be aware of the sensitivity of AI
Another aspect of generative models that contributes to the creation of AI hallucinations is the sensitivity and misinterpretation of the inputs or prompts they receive.
Generative models are sensitive to our word choice and how frame our requests.
A prompt that's missing a few keywords or contains some slang words that are easily understandable to the user might in turn cause the generative model to produce a piece of misinformation.
It's like when a person is asked a question and unknowingly misunderstands it but then proceeds to answer it anyway. Giving their thoughts, opinions, and recommended next steps on the topic you are talking about.
When this occurs it usually goes one of two ways. The information presented is readily accepted as factual by the person who asked the question or the questioner recognises the error and tries to remedy the situation by saying “No no you misunderstand me. Let me explain differently”.
It’s a common occurrence in everyday life that we readily accept and expect from people. However, we don’t expect that kind of behaviour from AI programmes and generative models due to our assumptions that machines can easily understand the requests that we give to them.
The truth of the matter is that if you are not crystal clear in your request for a generative model, you cannot be certain that you are going to get a factually correct answer. Even if you do that, you cannot be sure that the answer you received from them is even factually correct until you do some independent fact-checking yourself.
Why Do We Need To Careful About This?
Well put simply, we are starting to depend on AI and generative models. Whether it’s in our personal, academic, or professional lives, we are using AI to help speed up tasks and automate our workflows as much as we can.
This is so we can focus on other tasks that require more of our focus and time. The results of this are quite prominent (SOURCE). However, this dependency can have a detrimental effect on its users.
Let’s Imagine An AI Hallucination Scenario
Let’s use an example to explain this. You might ask a generative model like ChatGPT to list a series of the most up-to-date guidelines on how to implement EU GDPR practices into your business. It generates a list of recommendations and then you implement some of them.
A few months later there’s a data breach at your company and you are discussing with the relevant authorities about how you proceeded after discovering this. The authorities then state that you did not implement the correct practices in line with GDPR. Much to your shock and horror.
You look back at the chat history and you notice that ChatGPT has experienced an AI hallucination. It generated a list of recommendations that were a mix of US and EU data protection practices.
As a result of this, the company is now being fined thousands of euros and the headaches your company has to suffer have only increased.

What You Need To Acknowledge From This
Yes, this example is specific but it should give you an idea of what an AI hallucination can do to your business. The main takeaway you should get from this scenario is that companies, organisations, and even individuals have to accept generative models like ChatGPT are capable of mistakes.
If you are looking for factual information that is relevant to your needs, you should always make sure to fact-check afterward. The time it takes to fact-check an AI hallucination is so much smaller compared to dealing with the aftermath of one that gave you misinformation.
So How Do You Detect AI Hallucinations Then? Simple; Factiverse

Use Factiverse’s AI to Fact-check Text your Generative AI Model Produces
Luckily there are tools that allow you to fact-check more easily and Factiverse has some of the best tools available for that job.
Use Factiverse’s AI Editor to detect Hallucinations
First is Factiverse’s AI editor. It’s a simple tool that automates fact-checking for text that is produced from generative models like ChatGPT.
To use the AI editor all you have to do is copy and paste your content that was generated from the model you used into Factiverse AI Editor. It will then point out any hallucinations that your model may have experienced with sources to correct information about the claim the generative model generated.
Factiverse’s AI Editor searches through Google, Bing, and Semantic Scholar (200mil papers) simultaneously to find sources relevant to what your generative model has generated.
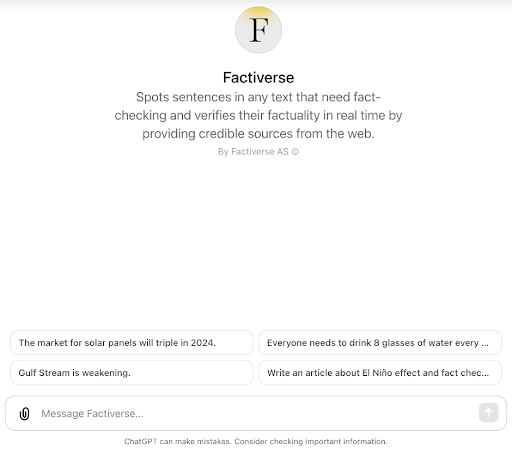
Add Factiverse’s ChatGPT to catch hallucinations in real time
To make the stamp out misinformation even easier, the AI Editor is also available as a plugin on Google Chrome and ChatGPT. Factiverse GPT can be used for instant and accurate fact-checking in over 40 languages. It quickly detects factual statements and cross-references them with the most credible real-time sources. You can literally detect any hallucinations in real time and save your own time fact-checking it later.
Here are short video demos of both products in action!
Referenced Sources:
.webp)